Staying informed about how apps collect and use your data is crucial in digital privacy. Addressing this, Appicaptor finds privacy-related app issues and extracts contents of the iOS Privacy Manifest. All information is correlated with results from static and dynamic analysis to provide enhanced privacy insights.
In today’s interconnected digital landscape concerns related to data privacy are in focus. App developers, platform providers and smartphone OS manufacturers face pressure to prioritize user privacy and transparency. In response to these concerns Apple has introduced an initiative: the iOS Privacy Manifest. This feature serves as a documentation how apps access, utilize and transmit privacy-related data. Appicaptor uses the information from the iOS Privacy Manifest, enabling users and companies to make well-informed decisions.
Understanding the iOS Privacy Manifest
iOS Privacy Manifests are attached to the app’s binary as property list (plist) and hold information regarding the collection and usage of privacy-related data by the app and included third-party SDKs. Not providing valid information within the iOS Privacy Manifest may lead to notifications or rejection throughout the app submission process. Strict enforcement in the App Store is scheduled starting in May 2024.
Privacy Manifest Components
When it comes to populating a iOS Privacy Manifest, there are several key data structures that developers must adhere to:
- Required Reason APIs: Apple introduced a class of APIs referred to as Required Reason APIs to address concerns regarding fingerprinting. Any app or SDK utilizing an API from this list must explicitly state a valid purpose for their usage.
- Data Usage Categories: Developers must provide a breakdown of data usage categories in their privacy manifests. This includes specifying the data collected, whether it’s linked to end-users’ identities, its usage for tracking purposes, and a list of reasons justifying. Apple provides a predefined set of purposes that developers have to reference.
- External Domain Usage: All external tracking domains used in the app or third-party SDKs must be listed in the iOS Privacy Manifest. This provides users with clear visibility into the presence of all domains utilized for tracking purposes. When tracking permission (via the App Tracking Transparency framework) is not granted by the user, network requests to these domains will be blocked by iOS.
Appicaptor: iOS Privacy Manifest as additional App Evaluation Source
Appicaptor extracts the contents of the iOS Privacy Manifest and presents them in human-readable format. But beyond that, Appicaptor correlates the manifest’s content with privacy-related findings discovered from the app binary through static analysis. Therefore, Appicaptor offers users a comprehensive understanding of how their privacy-related data is handled by the apps:
- Privacy Transparency: By presenting the iOS Privacy Manifest contents in a human-readable format, Appicaptor users gain transparency into the app’s data collection practices.
- Informed Decision-Making Elevated: Armed with in-depth understanding of an app’s privacy practices, Appicaptor users can make informed decisions about whether to engage with the app. Correlation of the manifest contents with static analysis findings in coordination with Appicaptor’s ability to define custom rulesets empowers companies to assess the privacy risks associated with using the app to make choices aligned with their privacy preferences and concerns.
- Enhanced Privacy Insights: Appicaptor’s static analysis enables an examination of the app’s codebase, revealing privacy insights in addition to what is disclosed in the iOS Privacy Manifest. By correlating these findings, users gain a more granular understanding of how their data is collected, used, and shared by the app.
- Accountability and Trust Building: Appicaptor’s evaluation reports promotes accountability and trust by ensuring alignment between stated privacy practices and actual implementation. By correlating the results, Appicaptor users can identify and address any discrepancies between the documentation and implementation of privacy-related data usage.
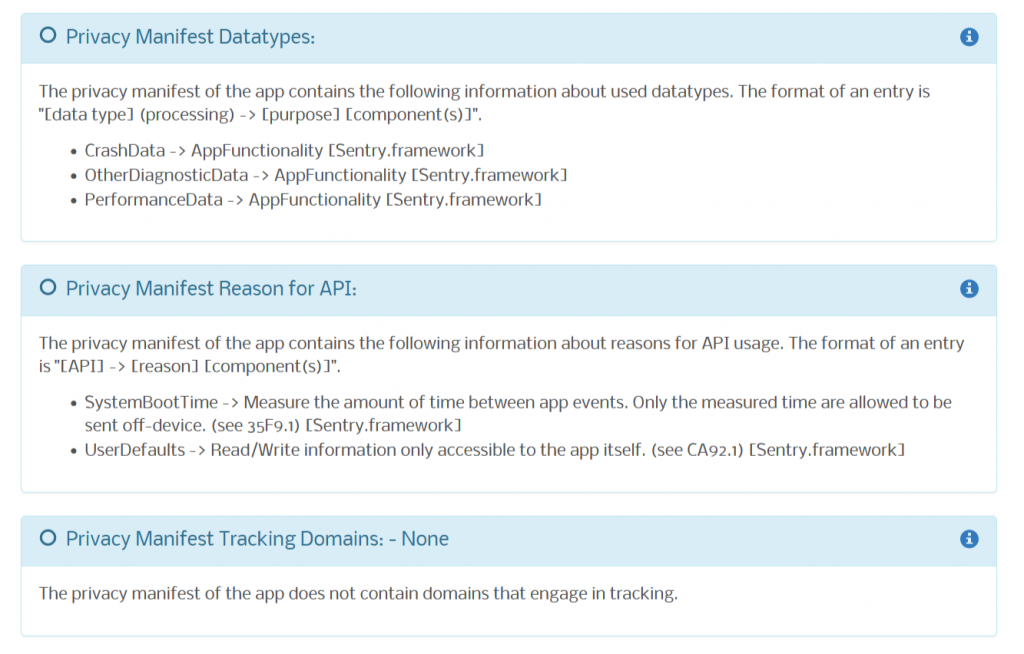
Conclusion
In an era where trust between users and tech companies is important, transparency regarding data collection practices is non-negotiable. Using the iOS Privacy Manifest as an additional source of evaluation, Appicaptor enhances its app evaluation capabilities and gives users a more thorough understanding of how their data is handled by various apps. This integration enforces Appicaptor’s commitment empowering users with transparent and informed decisions regarding app security.
Appicaptor’s solution contributes to the overall integrity of the digital ecosystem by promoting transparency, accountability, and responsible data usage. By empowering users with comprehensive privacy insights and encouraging developers to uphold best practices and that the implementation is inline with documentation, Appicaptor’s approach supports a culture of trust and integrity that benefits everyone involved.